Navigating the Data Science Learning Curve: A Journey of Discovery
Written on
Chapter 1: The Challenge of Learning
Embarking on the journey of learning something new can often feel overwhelming. There are moments when I receive inquiries about the process of acquiring knowledge, particularly in the field of data science. It's essential to clarify that I am not an authority on formal or informal education in this domain. Rather, I believe that individuals with experiences similar to mine might resonate with the insights I share, just as I once connected with the experiences of others.
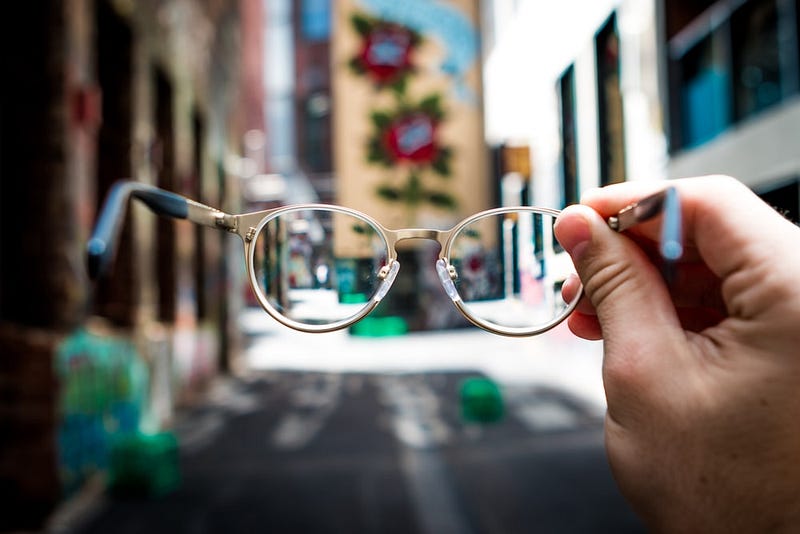
What potentially makes my reflections beneficial is my unconventional entry into the field of data science and artificial intelligence. Many are currently attempting to make a similar transition. This sector is undeniably appealing: it’s highly innovative, rapidly evolving, offers lucrative salaries, and plays a pivotal role in shaping the future. For some, data science or AI might represent a long-awaited opportunity—a domain that harmonizes various passions spanning mathematics, computer science, business, and marketing.
Speaking of marketing, I learned during my college years that it revolves around comprehending user behavior, a role that has recently shifted towards User Experience (UX). The correlation between data science and UX is evident; I suspect many are caught at the intersection of these fields, which likely explains the uptick in individuals seeking to transition into data-related careers.
I have a background in Business, and at one point, I began a Computer Science degree that I ultimately did not complete. Ten years ago in Uruguay, data wasn’t a prominent field, and I couldn't envision a complete shift into programming. Given my established career, I chose to remain focused on it. It was only three years ago that I contemplated pivoting into data science.
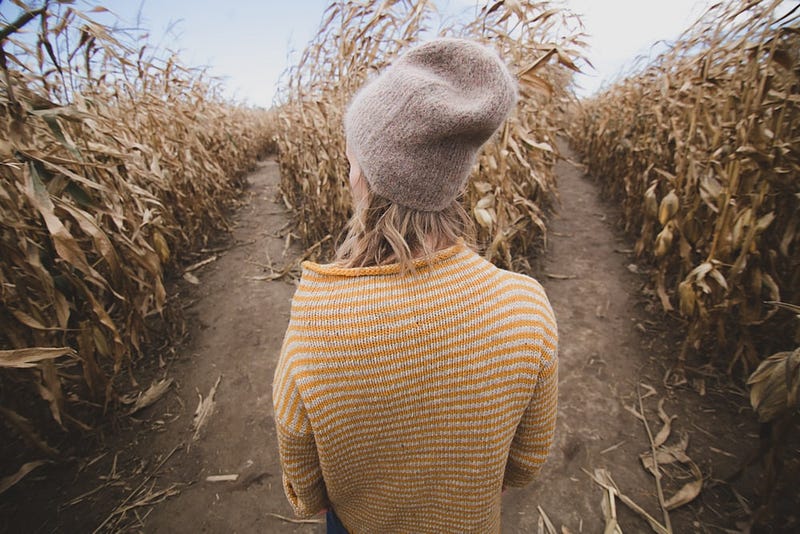
Like many others in this field, I've grappled with imposter syndrome since my first class. It’s a challenge that’s hard to evade, but I genuinely believe it can be mitigated by continuous self-improvement. This, of course, is no easy feat, especially for those of us coming from entirely different backgrounds. The prospect of an endless learning journey can be quite intimidating.
Now, three years into this adventure, with some valuable experience at Ravelin Technology and working on my own projects at Datahaus, I want to share some insights I've gained, particularly regarding my decision to pursue a master's degree and its progress.
Section 1.1: The Path Forward After Learning
After completing an introductory course in data science and acquiring some knowledge in Python and SQL, you might face the harsh reality that you are one among countless others on a similar path. While your previous experiences might give you an edge, as the field expands, so does the competition, leading to a diminishing chance of landing that first data science job. This realization often prompts significant questions: What should I learn next? Where should I begin?
Attempting to answer these questions, especially when transitioning from a different background and lacking relevant experience, can be daunting. Investing time in the wrong subjects can lead to wasted effort and frustration. I often found myself in courses that required prior knowledge I simply didn’t have—an experience many can relate to.
It's not just about Mathematics, Statistics, Computer Science, and Business. Simplifying the foundations of this field to just those topics overlooks its complexity. For instance, Computer Science encompasses much more than mere programming. It includes concepts such as programming paradigms, object-oriented programming, functional programming, and algorithm analysis. Similarly, Mathematics and Statistics involve intricate areas like logic, calculus, and probability, each with their own expansive subtopics. Even Artificial Intelligence has its unique core concepts, such as logical reasoning and decision processes.
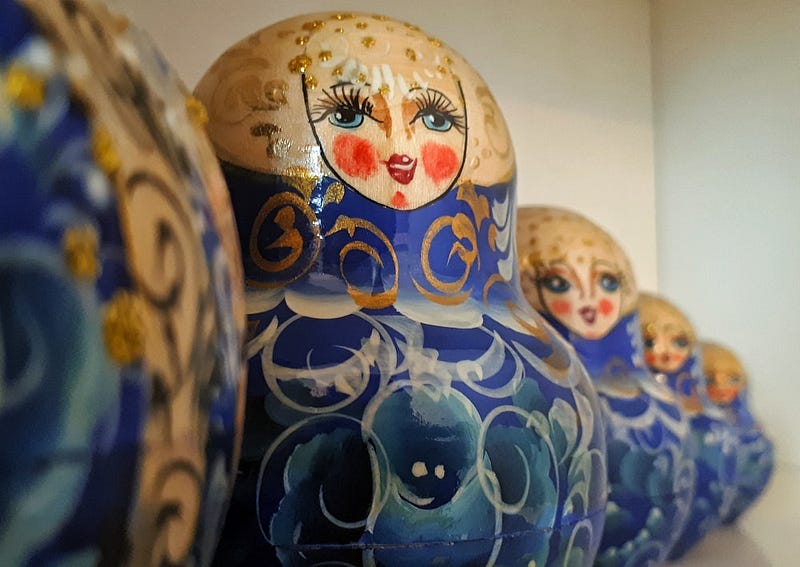
A set of matryoshkas consists of a wooden figure, which separates at the middle, top from bottom, to reveal a smaller figure of the same sort inside, which has, in turn, another figure inside of it, and so on.
So here I am, fresh from a Data Science bootcamp, pondering my next steps without having a clear direction. This uncertainty led me to enroll in a master's program; I recognized that I needed guidance.
Not only had I spent time on courses that didn't align with my knowledge level, but I also found myself learning from the wrong sources. The realm of data science and AI is rich with online resources, but it’s crucial to discern between quality content and misinformation. While there are excellent courses available, choosing the right instructor is vital. A course’s effectiveness is often contingent on the expertise of its educators.
Moreover, the phenomenon of choice overload can complicate the decision-making process, making it challenging to select the best learning path.
Section 1.2: Weighing the Pros and Cons of Further Education
However, pursuing a master's degree comes with its own set of challenges, including a lack of flexibility. If you urgently need to learn about specific technologies like Kubernetes or Docker, you might find yourself instead immersed in abstract discussions on intelligence concepts. Additionally, the financial implications are significant. Yet, if you can manage the investment and, like me, feel lost regarding the next steps, hiring an expert to guide you can be beneficial, helping to identify not only topics but also valuable resources.
Ultimately, this journey can be viewed as an optimization problem, balancing actions, probabilities, and potential rewards. Interestingly, I didn’t formally understand optimization problems until recently. Now, I find myself contemplating how to frame this situation as a Markov Decision Process, even if I am not quite there yet—perhaps in the future!
Chapter 2: Insights from Experience
This video explores key takeaways from a recent learning experience, highlighting the importance of continuous learning in the field of data science.
In this video, we discuss practical steps for transitioning into data science, including tips for navigating the vast array of learning resources available online.